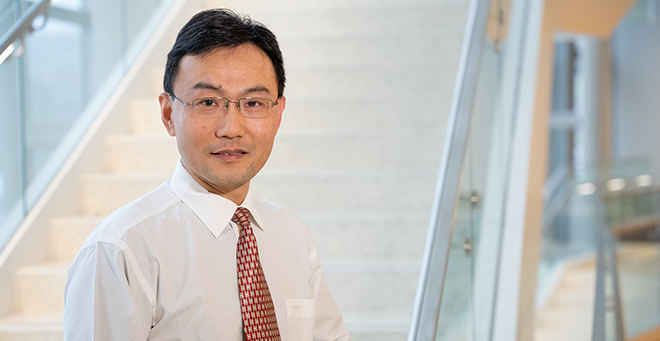
Photo: Bryan Goodchild
Artificial intelligence (AI) is already changing the way health care is provided, with enhanced diagnostic accuracy of images, predictive analyses of patient outcomes from large data sets that can direct treatment plans and analyzing individual patient data to tailor interventions to personal needs.
UMass Chan Medical School researcher Feifan Liu, PhD, associate professor of population & quantitative health sciences, is part of a national effort to spearhead another important application of AI: to advance health equity.
In 2022, Dr. Liu was among the first cohort of leadership fellows in the National Institutes of Health’s Artificial Intelligence/Machine Learning Consortium to Advance Health Equity and Research Diversity (AIM-AHEAD) program, a partnership to enhance participation and representation of researchers and communities underrepresented in the development of AI and machine learning models, and to improve the capabilities of this emerging technology to address health disparities and inequities.
“Feifan’s work with AIM-AHEAD and being part of this AIM-AHEAD structure is leading the way in terms of thinking, not just what the value is of AI, but how does this work in the health care system and society at large?” said Ben S. Gerber, MD, MPH, professor of population & quantitative health sciences, who works with Liu on several projects. “What are the risks? How do we deal with fairness, bias and trust, and other ethical issues of artificial intelligence?”
Liu is principal investigator on two major research initiatives that grew out of the AIM-AHEAD fellowship. The first, DETERMINE (Diabetes Prediction and Equity through Responsible Machine Learning), is a $1.4 million, two-year NIH AIM-AHEAD consortium development grant in partnership with University of Illinois Chicago and Temple University to develop an AI-powered multivariable risk prediction model to integrate social, demographic and clinical factors for accurate, fair, generalizable and interpretable type 2 diabetes prediction. Dr. Gerber is co-principal investigator of the study, now in its second year.
“The main goal is to build a responsive AI model predicting the risk of developing type 2 diabetes and evaluate how well the model generalizes across different institutions as well as how equitably the model performs across different demographic subgroups,” said Liu. “We will also conduct simulation analyses and illustrate the potential impact on real-world clinical practice, and improving access to preventive medicine or prevention programs, especially for minority groups disproportionately affected by type 2 diabetes.”
The heart of AI applications is the algorithms on which machine learning models are based.
Existing clinical guidelines for type 2 diabetes preventive measures rely on a simplified, imprecise prediabetes definition, which relies on limited measures such as glycemia and body mass index, Liu explained. The researchers are integrating nonmedical socioeconomic data involving neighborhood, environment and economic characteristics into the DETERMINE algorithm that they hope will more accurately identify people at risk and lead to more equitable distribution of prevention and treatment resources.
The second study, AI2Equity, is a $3 million, four-year grant funded by the National Heart, Lung and Blood Institute in 2024. In partnership with OCHIN, a national community health network, and Temple University, the multidisciplinary team of researchers aims to build a deep learning model incorporating social determinants of health, structured electronic health records and clinical notes to improve prediction of cardiovascular disease. The project provides a solid foundation for advancing equitable cardiovascular disease prevention, according to Liu.
The model will be compared with currently used cardiovascular risk prediction tools.
“For both projects, we will assess and improve the generalizability and model fairness across different institutions and different settings,” Liu said. “To mitigate bias, we will develop training algorithms that ensure model training excludes information closely linked to sensitive attributes such as race or ethnicity. Studies show that AI could unintentionally amply signals from bias data, exacerbating disparities for marginalized groups. Finally, we want to show that the model has better interpretability to better support clinical decision making.”
Liu and Gerber said that the sooner and more accurately the risk of developing diabetes or cardiovascular disease can be identified, the better the health outcome—preventing or delaying disease with lifestyle and medication therapy.